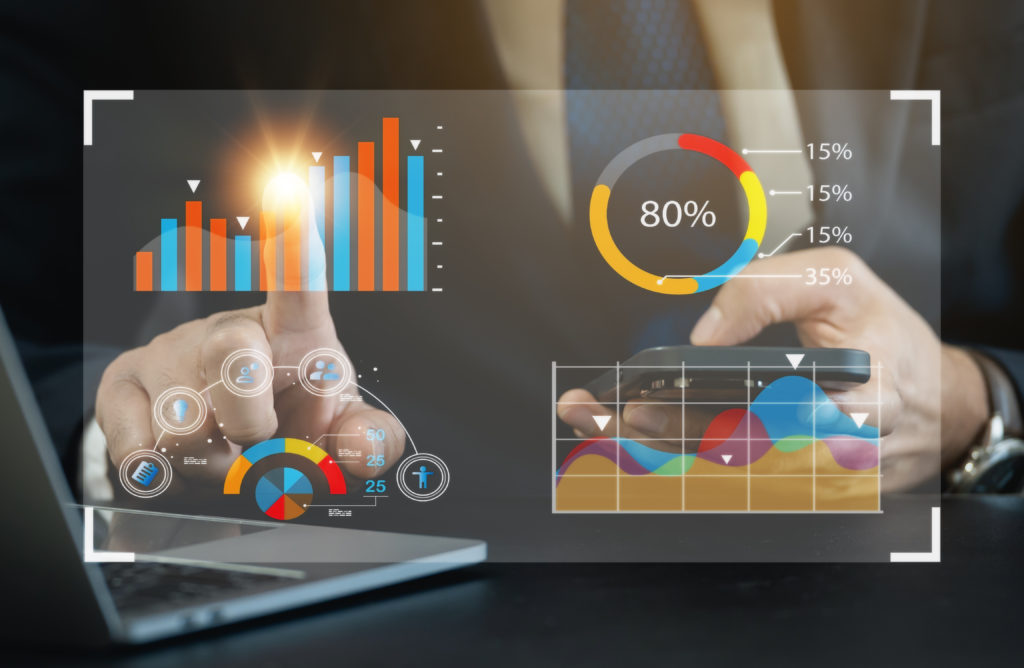
Image Source: FreeImages
Introduction to data analytics
Data analytics has become an integral part of modern businesses, providing valuable insights and guiding strategic decision-making. In today’s data-driven world, organizations are increasingly relying on data to gain a competitive edge. In this article, we will delve into the different types of analytics and how they contribute to business success.
The importance of data analytics for businesses
Data analytics plays a crucial role in helping businesses make informed decisions and identify patterns and trends that may otherwise go unnoticed. By leveraging the power of data, organizations can gain a deep understanding of their customers, optimize their operations, and uncover new growth opportunities.
One of the key benefits of data analytics is the ability to enhance customer experience. By analyzing customer data, businesses can gain insights into customer preferences, behavior patterns, and pain points. This information can be used to personalize marketing campaigns, improve product offerings, and deliver exceptional customer service.
Furthermore, data analytics enables organizations to optimize their operations and increase efficiency. By analyzing internal data, businesses can identify bottlenecks, streamline processes, and make data-driven improvements. This not only leads to cost savings but also improves overall productivity and performance.
Exploring different types of analytics
Data analytics can be broadly categorized into four main types: descriptive analytics, diagnostic analytics, predictive analytics, and prescriptive analytics. Each type serves a unique purpose and provides specific insights to support decision-making.
a. Descriptive analytics
Descriptive analytics focuses on summarizing historical data to understand what has happened in the past. It involves analyzing data to uncover patterns, trends, and relationships. This type of analytics provides a snapshot of the current state of affairs and is often used to generate reports and dashboards.
For example, a retail company may use descriptive analytics to analyze sales data from the past year and identify the best-selling products, peak sales periods, and customer buying patterns. This information can help the company make data-driven decisions related to inventory management, pricing, and marketing strategies.
b. Diagnostic analytics
Diagnostic analytics goes a step further than descriptive analytics by seeking to understand why something has happened. It involves drilling down into the data to identify the root causes of a particular outcome or event. Diagnostic analytics helps businesses gain insights into the factors that influence their performance.
Continuing with the previous example, the retail company may use diagnostic analytics to investigate a sudden drop in sales during a specific period. By analyzing additional data such as customer feedback, competitor analysis, and market trends, the company can identify the reasons behind the decline and take corrective actions accordingly.
c. Predictive analytics
Predictive analytics takes data analysis to the next level by using historical data to make predictions about future outcomes. By applying statistical models and machine learning algorithms, businesses can forecast trends, anticipate customer behavior, and make proactive decisions.
For instance, an e-commerce company can use predictive analytics to analyze past purchase behavior and predict which customers are most likely to make a repeat purchase. This information can be used to target these customers with personalized offers and recommendations, increasing the chances of conversion and customer loyalty.
d. Prescriptive analytics
Prescriptive analytics is the most advanced form of data analytics. It not only predicts future outcomes but also provides recommendations on the best course of action to achieve a desired outcome. This type of analytics is particularly useful in complex decision-making scenarios.
Consider a transportation company that wants to optimize its delivery routes to minimize fuel consumption and maximize efficiency. By using prescriptive analytics, the company can analyze various factors such as traffic patterns, delivery volumes, and vehicle capacities to determine the most optimal routes and schedules.
Real-life examples of data analytics in action
To better understand the practical applications of data analytics, let’s explore some real-life examples of how businesses have leveraged data to drive success.
Example 1: Netflix
Netflix, the leading streaming platform, extensively uses data analytics to personalize user recommendations and enhance the overall viewing experience. By analyzing user behavior, Netflix can recommend personalized content based on a user’s viewing history, ratings, and preferences. This not only improves customer satisfaction but also increases user engagement and retention.
Example 2: Amazon
Amazon, the world’s largest e-commerce platform, relies heavily on data analytics to optimize its supply chain and improve customer satisfaction. By analyzing customer data, Amazon can anticipate customer demand, optimize inventory levels, and provide accurate delivery estimates. This enables Amazon to deliver a seamless shopping experience and maintain its position as the market leader.
Example 3: Uber
Uber, the popular ride-hailing service, utilizes data analytics to optimize its pricing strategies and driver allocation. By analyzing data such as demand patterns, traffic conditions, and driver availability, Uber can dynamically adjust prices to match supply and demand. This ensures efficient utilization of drivers and enhances the overall customer experience.
Implementing data analytics in your business
Now that we understand the different types of analytics and their applications, let’s explore how you can implement data analytics in your own business.
a. Setting goals and objectives
Before diving into data analytics, it is crucial to define clear goals and objectives. Determine what insights you want to gain from the data and how they align with your business objectives. This will help guide your data analysis efforts and ensure that you are focusing on the right metrics.
b. Collecting and organizing data
Once you have defined your goals, the next step is to collect and organize relevant data. This may involve integrating data from multiple sources, such as customer databases, sales records, and website analytics. It is essential to ensure the accuracy and quality of the data to derive meaningful insights.
c. Analyzing and interpreting data
After collecting the data, it’s time to analyze and interpret it. This can be done using various statistical techniques, data visualization tools, and machine learning algorithms. The goal is to uncover patterns, identify trends, and gain actionable insights that can drive decision-making.
d. Making data-driven decisions
The final step in implementing data analytics is using the insights gained to make data-driven decisions. This requires translating the analysis into actionable strategies and initiatives. It is important to communicate the findings to key stakeholders and ensure that the organization embraces a data-driven culture.
Tools and software for data analytics
To effectively implement data analytics, businesses can leverage a wide range of tools and software. Here are some popular options:
- Tableau: A powerful data visualization tool that allows users to create interactive dashboards and reports.
- Google Analytics: A web analytics tool that provides valuable insights into website traffic and user behavior.
- Python: A popular programming language for data analysis, with libraries such as Pandas and NumPy.
- R: A statistical programming language commonly used for data analysis and visualization.
- SAS: An advanced analytics software suite that offers a wide range of tools for data exploration, modeling, and reporting.
Choosing the right tools depends on your specific requirements, budget, and technical expertise. It is advisable to evaluate multiple options and select the ones that best suit your needs.
Challenges and considerations in data analytics
While data analytics offers immense benefits, it also comes with its own set of challenges and considerations. Here are some key factors to keep in mind:
- Data quality: The accuracy, completeness, and consistency of data are critical for meaningful analysis. Ensuring data quality requires proper data governance and data cleansing processes.
- Data privacy and security: With the increasing volume of data being collected, organizations need to prioritize data privacy and security. Implementing robust security measures and complying with relevant regulations is essential.
- Skill gap: Data analytics requires a combination of technical and analytical skills. Finding skilled data analysts or upskilling existing employees can be a challenge for organizations.
- Data integration: Integrating data from various sources and formats can be complex. Organizations need to invest in proper data integration tools and processes to ensure seamless data flow.
- Ethical considerations: Data analytics raises ethical questions around privacy, bias, and transparency. Organizations need to establish ethical guidelines and ensure responsible use of data.
Hiring data analysts or outsourcing analytics services
Depending on the size and complexity of your business, you may consider hiring data analysts or outsourcing analytics services. Hiring data analysts allows you to build an in-house team with deep expertise in data analytics. On the other hand, outsourcing analytics services to specialized firms can provide cost-effective solutions and access to advanced analytics capabilities.
When making a decision, consider factors such as budget, long-term goals, and the availability of skilled resources. Both options have their own pros and cons, so it is important to evaluate which approach aligns best with your business strategy.
Case studies of successful data analytics implementation
To further illustrate the impact of data analytics, let’s delve into a couple of case studies showcasing successful implementation.
Case Study 1: Walmart
Walmart, the multinational retail corporation, leverages data analytics to optimize its supply chain and inventory management. By analyzing sales data, weather patterns, and historical trends, Walmart can accurately forecast demand and adjust inventory levels accordingly. This has resulted in significant cost savings and improved customer satisfaction.
Case Study 2: Starbucks
Starbucks, the global coffeehouse chain, uses data analytics to personalize the customer experience and drive customer loyalty. By analyzing customer preferences, purchase history, and location data, Starbucks can offer personalized recommendations and promotions through its mobile app. This has led to increased customer engagement and higher sales.
Conclusion: Harnessing the power of data for business success
In conclusion, data analytics is a powerful tool that can unlock valuable insights and drive business success. By adopting a data-driven approach, organizations can make informed decisions, optimize operations, and deliver exceptional customer experiences. From descriptive analytics to prescriptive analytics, the different types of analytics offer a range of capabilities to suit various business needs. Implementing data analytics requires clear goals, quality data, analytical expertise, and the right tools. By overcoming challenges and leveraging the power of data, businesses can gain a competitive edge and thrive in today’s data-driven world. So, embrace the power of data analytics and unlock the potential for business success.
CTA: Start harnessing the power of data analytics in your business today and unlock new opportunities for growth and success.