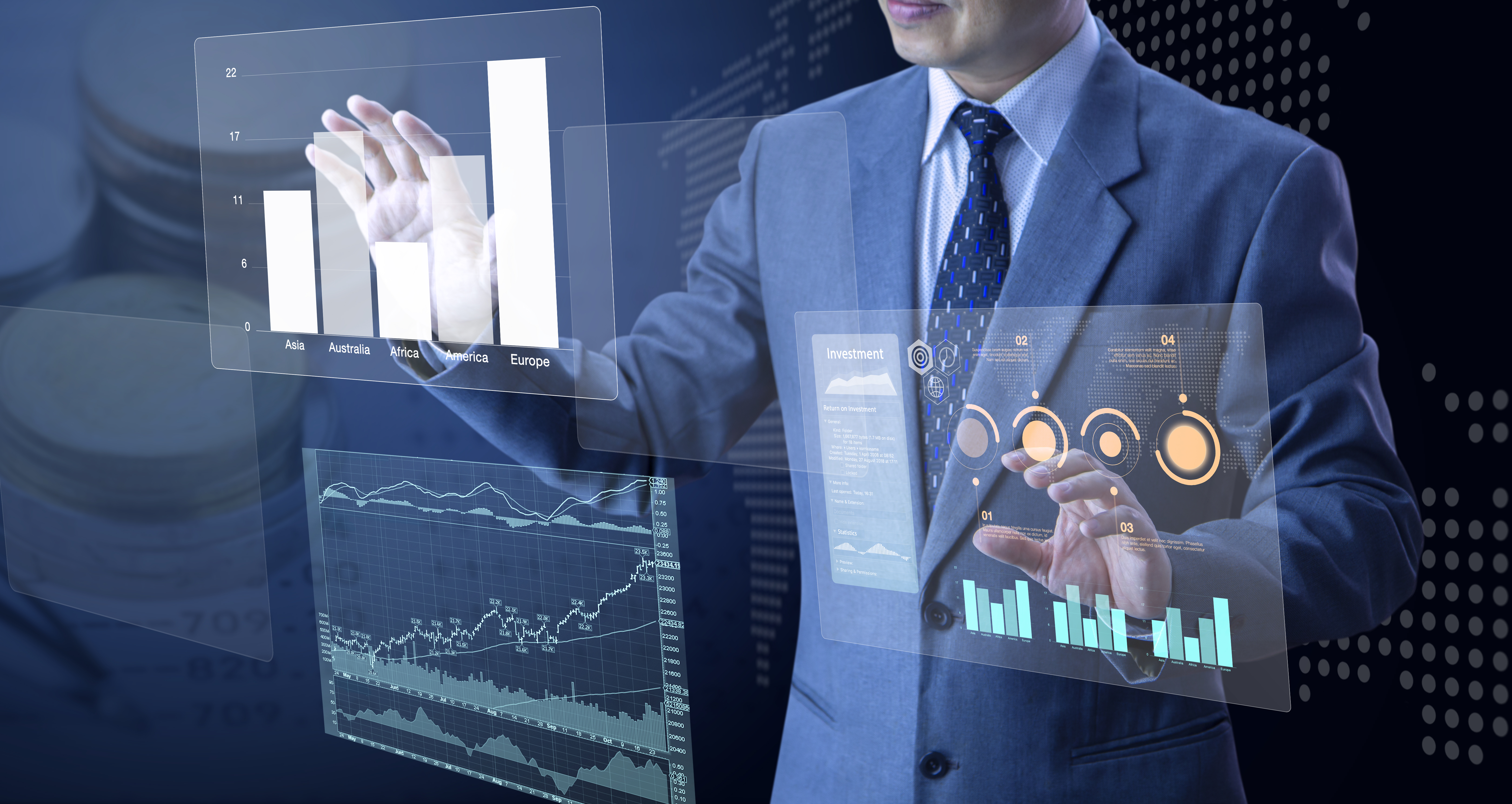
Image Source: FreeImages
Introduction to Data Analytics
In today’s digital age, organizations are inundated with a vast amount of data pouring in from various sources. This data holds the key to valuable insights that can drive informed decision-making and improve business outcomes. However, the sheer volume and complexity of data can be overwhelming. This is where data analytics comes into play. Data analytics is the process of examining raw data to uncover hidden patterns, correlations, and trends. By harnessing the power of data analytics, organizations can unlock valuable insights that can revolutionize their operations, drive innovation, and gain a competitive edge.
Why Data Analytics is Important
Data analytics is crucial for organizations across industries due to its ability to transform raw data into actionable insights. By analyzing data, organizations can gain a deeper understanding of customer behavior, identify market trends, optimize operations, and predict future outcomes. With the right insights, organizations can make data-driven decisions that lead to improved efficiency, reduced costs, increased profitability, and enhanced customer satisfaction. In today’s highly competitive business landscape, data analytics has become a strategic imperative for organizations seeking to stay ahead of the curve and thrive in the digital era.
Types of Data Analytics
Data analytics encompasses various techniques and methodologies that can be classified into different types based on the objectives and approaches. The three main types of data analytics are descriptive analytics, predictive analytics, and prescriptive analytics.
Descriptive Analytics: Descriptive analytics focuses on summarizing historical data to provide insights into past events and trends. It helps organizations understand what happened and why it happened by examining patterns, frequencies, and distributions within the data.
Predictive Analytics: Predictive analytics leverages historical data and statistical modeling techniques to make predictions about future events or outcomes. By identifying patterns and correlations, predictive analytics enables organizations to anticipate trends, forecast demand, and mitigate risks.
Prescriptive Analytics: Prescriptive analytics goes beyond descriptive and predictive analytics by providing recommendations and actionable insights. It uses optimization algorithms and simulation techniques to determine the best course of action to optimize outcomes and achieve desired goals.
The Data Analytics Process
The data analytics process consists of several interrelated stages that guide organizations in extracting insights from data. These stages include data collection, data cleaning and preparation, data analysis, and data interpretation.
Data Collection: The first step in the data analytics process is collecting relevant data from various sources such as databases, websites, social media, and sensors. This data can be structured or unstructured, and it may come in different formats like text, numbers, images, or videos.
Data Cleaning and Preparation: Once the data is collected, it needs to be cleaned and prepared for analysis. This involves removing duplicates, handling missing values, transforming data into a consistent format, and ensuring data quality and integrity.
Data Analysis: After data cleaning, the next step is to analyze the data using statistical techniques, machine learning algorithms, and data mining methods. This involves exploring the data, identifying patterns and trends, and applying appropriate models to extract meaningful insights.
Data Interpretation: The final stage of the data analytics process is interpreting the results and translating them into actionable insights. This requires domain expertise, critical thinking, and effective communication skills to convey the findings to stakeholders and decision-makers.
Tools and Technologies for Data Analytics
Data analytics requires the use of various tools and technologies to effectively analyze and interpret data. These tools range from data management and processing platforms to advanced analytics software and visualization tools.
Data Management and Processing Platforms: Data management platforms like Apache Hadoop and Apache Spark are widely used for storing and processing large volumes of data. They provide scalable and distributed computing capabilities, enabling organizations to handle big data efficiently.
Advanced Analytics Software: Advanced analytics software such as Python, R, and SAS are popular choices for performing complex data analysis tasks. These tools offer a wide range of statistical and machine learning algorithms, making it easier to extract insights from data.
Visualization Tools: Data visualization tools like Tableau, Power BI, and QlikView are essential for presenting data in a visually appealing and intuitive manner. They enable analysts to create interactive charts, graphs, and dashboards that facilitate better understanding and decision-making.
Data Visualization and Storytelling
Data visualization plays a crucial role in data analytics as it helps to communicate insights effectively. By transforming complex data into visual representations such as charts, graphs, and infographics, data visualization enhances understanding, reveals patterns, and supports decision-making.
Effective data visualization goes beyond creating visually appealing charts. It involves storytelling – using data to tell a compelling narrative that engages the audience and conveys the insights in a meaningful way. By combining data, visuals, and storytelling techniques, organizations can create a powerful tool for communicating insights and driving action.
Applications of Data Analytics in Different Industries
Data analytics finds applications in a wide range of industries, revolutionizing operations and driving innovation.
Retail and E-commerce: Data analytics helps retailers analyze customer purchasing patterns, optimize inventory management, personalize marketing campaigns, and predict demand, leading to increased sales and customer satisfaction.
Finance and Banking: In the finance industry, data analytics is used for fraud detection, risk assessment, credit scoring, portfolio optimization, and personalized financial advice.
Healthcare: Data analytics is transforming healthcare by improving patient care, optimizing treatment plans, predicting disease outbreaks, and facilitating medical research and development.
Manufacturing: In manufacturing, data analytics enables predictive maintenance, quality control, supply chain optimization, and process optimization, resulting in reduced downtime and improved productivity.
Marketing and Advertising: Data analytics empowers marketers to analyze customer behavior, target specific segments, optimize advertising campaigns, and measure the effectiveness of marketing initiatives.
Challenges and Limitations of Data Analytics
While data analytics offers immense potential, it also comes with challenges and limitations that organizations need to address.
Data Quality and Integrity: Poor data quality, incomplete data, or biased data can lead to inaccurate insights and flawed decision-making. It is crucial to ensure data quality and integrity to achieve reliable results.
Data Privacy and Security: With the increasing amount of data being collected, organizations need to prioritize data privacy and security to protect sensitive information and comply with regulations.
Skill Gap: Data analytics requires a combination of technical skills, domain expertise, and business acumen. Organizations need to invest in training and hiring skilled professionals to build a successful data analytics team.
Ethical Considerations: Data analytics raises ethical concerns around privacy, bias, and fairness. Organizations must ensure that data analytics is conducted in an ethical and responsible manner to avoid negative consequences.
How to Build a Successful Data Analytics Team
Building a successful data analytics team requires careful planning and consideration. Here are some key steps to follow:
Define Objectives and Roles: Clearly define the objectives and goals of the data analytics team and identify the roles and responsibilities of team members.
Hire Skilled Professionals: Recruit talented individuals with a strong background in data analytics, statistics, programming, and domain knowledge.
Promote Collaboration: Foster a culture of collaboration and knowledge sharing within the team to leverage the diverse skills and expertise of team members.
Provide Training and Development: Invest in training and development programs to enhance the skills and capabilities of the team members and keep them updated with the latest tools and techniques.
Encourage Continuous Learning: Encourage team members to stay curious and explore new technologies and methodologies to stay ahead in the rapidly evolving field of data analytics.
Future Trends in Data Analytics
The field of data analytics is constantly evolving, driven by advancements in technology and the increasing availability of data. Here are some future trends that are shaping the future of data analytics:
Artificial Intelligence and Machine Learning: The integration of artificial intelligence and machine learning algorithms is revolutionizing data analytics by enabling automated insights, predictive modeling, and intelligent decision-making.
Real-time Analytics: Real-time analytics allows organizations to analyze data as it is generated, enabling proactive decision-making and immediate response to changing conditions.
Augmented Analytics: Augmented analytics combines human expertise with machine learning algorithms to automate data preparation, analysis, and interpretation, making data analytics more accessible to a wider range of users.
Internet of Things (IoT) Analytics: With the proliferation of IoT devices, organizations can collect and analyze real-time data from connected devices, enabling new insights and opportunities for innovation.
Ethics and Responsible Data Analytics: As data analytics becomes more pervasive, there is a growing emphasis on ethical considerations, transparency, and responsible use of data.
Conclusion
Data analytics has emerged as a game-changer for organizations, unlocking valuable insights from the vast amounts of data available today. By leveraging data analytics tools, techniques, and methodologies, organizations can gain a competitive edge, drive innovation, and make informed decisions that lead to improved business outcomes. However, it is important to address the challenges and limitations associated with data analytics and build a successful data analytics team to maximize the potential of data analytics. As the field of data analytics continues to evolve, embracing future trends and ethical considerations will be crucial for organizations to stay ahead and harness the power of data analytics for success.
Call to Action: Embrace the power of data analytics and unlock valuable insights for your organization. Invest in the right tools, technologies, and skilled professionals to build a successful data analytics team. Stay ahead of the curve by embracing future trends and ethical considerations in data analytics. Start your data analytics journey today and unleash the power of data!